In Full Bloom: Predicting HAB events using A.I.
By. Mia N. Judicpa & Gabrielle A. Abrahan, MSI Contributors
In 1983, many inhabitants of Samar, Masbate, Sorsogon, and even Western Visayas were gripped by symptoms like tingling, numbness, and nausea. Almost 300 cases of food poisoning were reported. The culprit was eventually identified to be the dinoflagellate Pyrodinium bahamense that affected green mussels in Maqueda Bay and Villareal Bay, where several mariculture facilities for shellfish were located. Shellfish harvesting was banned in those locations for eight months.1
Numerous reports of harmful algal bloom (HAB) events in the Philippines have surfaced since then. HABs are familiar to many of us — we know it as the “red tide,” a phenomenon whereby the open water becomes discolored, possibly in reddish hues, due to the overgrowth of algae. Still, the term “HAB” is not limited to describing red discoloration. Algae can turn the water yellow, blue, or even milky.
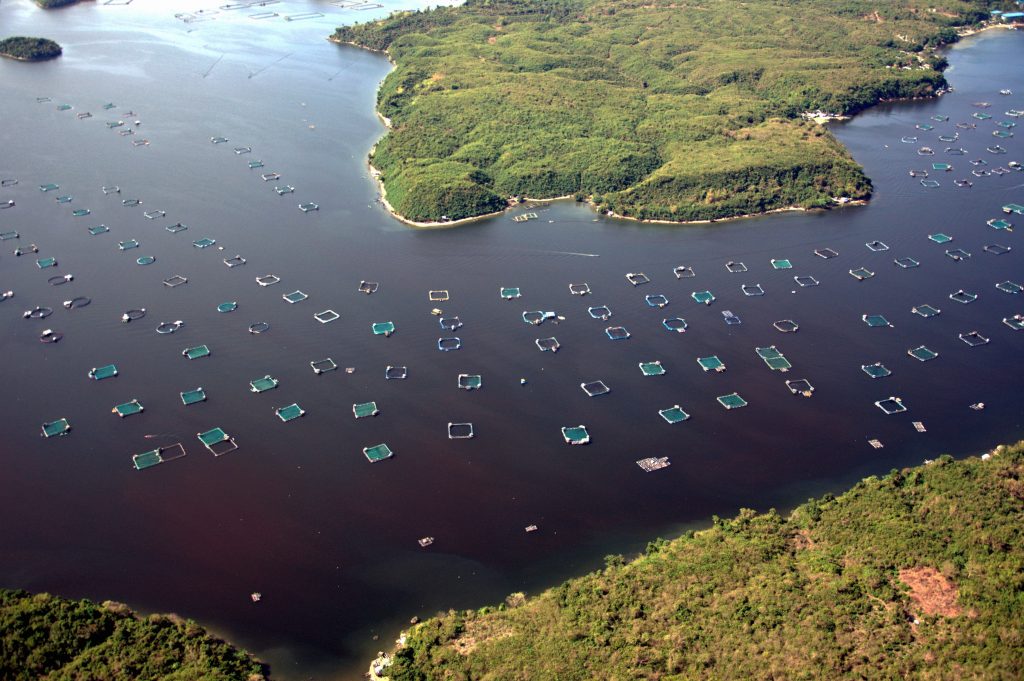
Photo: Dr. Gil Jacinto
Marine biologists are always on the lookout for HABs because of their associated threats. The algae may harbor toxins, as evidenced by the Paralytic Shellfish Poisoning (PSP) terror of 1983, or cause physical damage merely due to overgrowth.
Live tracking of HABs can be expensive, so Dr. Aletta Yñiguez and co-author Zheina Ottong turned to artificial intelligence (AI) to forecast these occurrences. “Predicting fish kills and toxic blooms in an intensive mariculture site in the Philippines using a machine learning model”2 presents promising results obtained through the random forest algorithm. The AI was proven to accurately identify fish kills and toxic blooms, giving us a headstart on achieving real-time monitoring of HABs.
Mysterious slurries
When a marine, freshwater, or estuary environment is overwhelmed by an algal bloom, it turns into a large body of sludge or foam. The process begins when algae cysts emerge from dormancy in the benthic region and germinate. These cysts hatch under the right conditions, releasing cells that reproduce continuously for weeks until they have proliferated enough to form the “bloom.”
Algal blooms appear different depending on the algae that cause them. The first recorded PSP event caused by P. bahamense was a red tide. On the other hand, algal blooms caused by cyanobacteria (blue-green algae) appear greenish. There are also bioluminescent algae that can illuminate seawater at night, shining blue light from below the water surface.
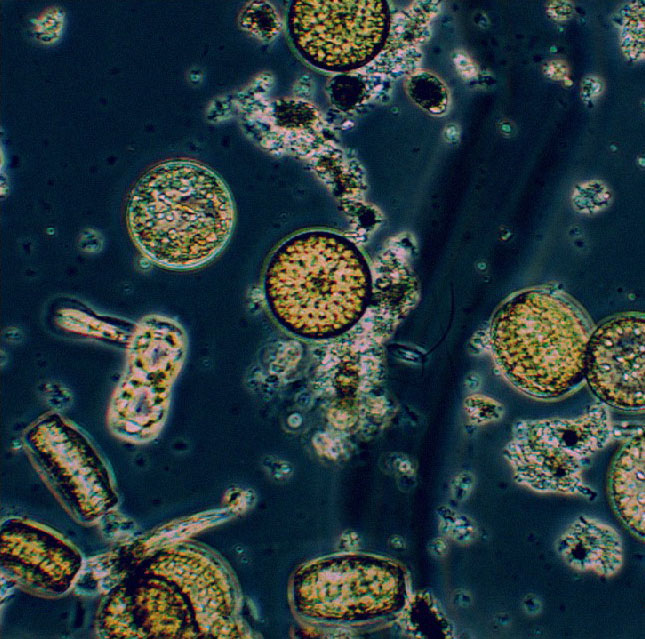
Photo: Jennifer Maister of UP MSI BiOME lab.
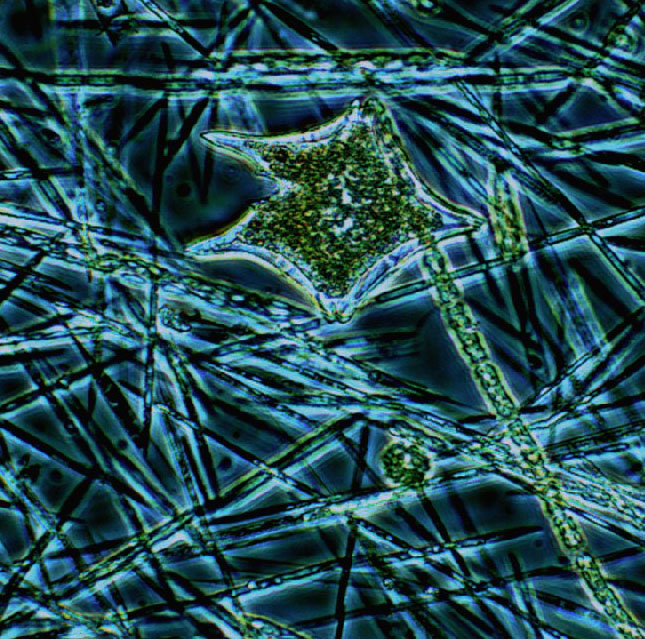
Photo: Jennifer Maister of UP MSI BiOME lab
Not all algal blooms are “harmful” — to be “harmful,” the algae in question must produce toxins. But non-toxic blooms are also dangerous because they produce large amounts of biomass. These are unwelcome changes that may harm the organisms sharing that habitat. Algal blooms dominate and compete with the natural phytoplankton population, leading to the collapse of the balance in the local ecosystem. Moreover, algal blooms lead to decreased dissolved oxygen levels, suffocating the fish population and causing fish kills.
Video: Rica Dungog of UP MSI BiOME lab
While regarded as threats, HABs actually occur naturally every year. They are not necessarily man-made phenomena; they have existed long before the first mention of climate change. In fact, HAB-causing species have been observed in fossil records. Certain conditions can increase the likelihood of a HAB happening, like high water temperature, slow water flow, and changes in the local ecology of a body of water.
The number one contributing factor is higher nutrient (phosphorus and/or nitrogen) levels. Algae are plants, and these nutrients serve as fertilizer to them. Human activities like agriculture, manufacturing, and sewage disposal increase nutrient levels in the water, and may therefore contribute to the spread and frequency of HAB phenomena. Transporting affected organisms to different locations (via trade) also helps spread HAB-causing algae.
Endangered livelihoods
HABs are a dire concern for many sectors of society — public health, economy, and livelihoods — and the development of early-warning tools can greatly improve our chances of mitigating them.
Many countries are actually regularly affected by HABs, but a growing body of evidence shows that these phenomena have been spreading. A recently published UN Global HAB Status Report on algal blooms3 found that the Philippines, a maritime country, experienced the highest number of PSP cases (around 67% of the global count) from 1985 to 2018, with 165 fatalities.
In the past year alone, several Philippine regions were affected by the red tide, including Palawan, Bohol, Negros Oriental, Western Samar, Leyte, Biliran, Davao Oriental, Surigao del Sur, and Zamboanga del Norte. Shellfish bans are enacted whenever needed.
“Usually, toxic blooms happen sa mga embayment like Manila Bay, Sorsogon Bay. Iyon ‘yung mga usual [spot]. Parating marami sa Visayas. Marami tayong bays eh, mga hotspot areas sa Visayas. In Mindanao now, medyo marami na rin naapektuhan,” Dr. Yñiguez explains when asked about the vulnerability of Philippine areas to HABs.
(“Usually, toxic blooms happen in embayments like Manila Bay, Sorsogon Bay. Those are the usual spots. There’s always a lot in the Visayas. We have numerous bays, hotspot areas in the Visayas. In Mindanao now, many areas are affected.”)
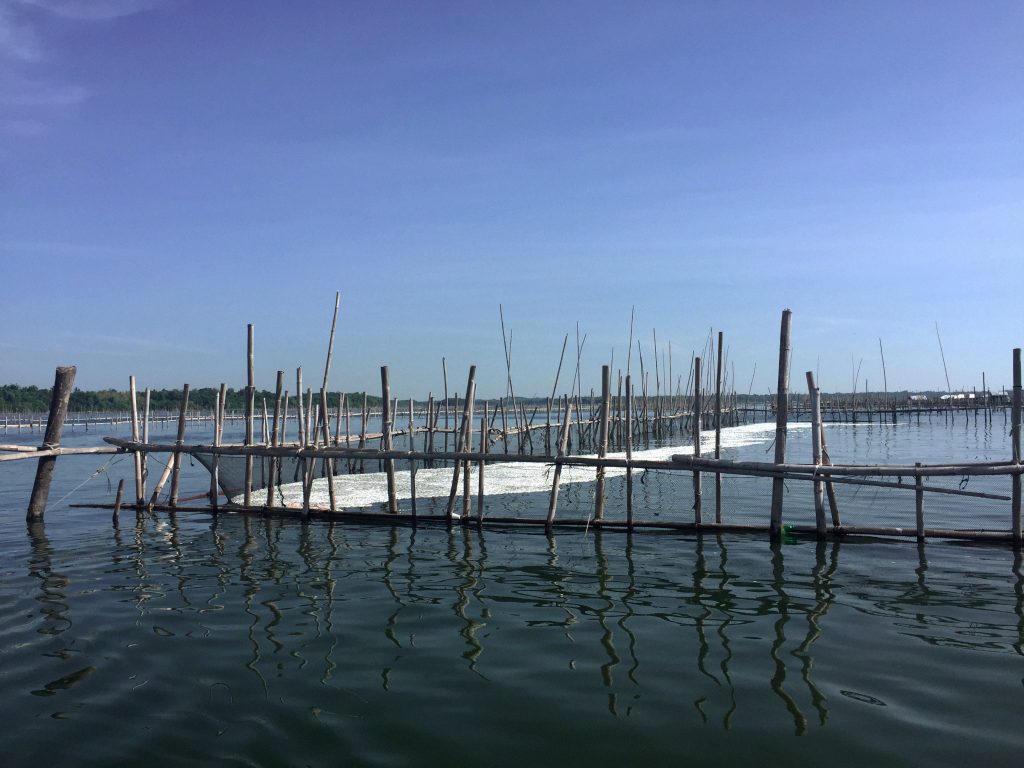
Photo: Rica Dungog of UP MSI BiOME lab
Food safety risk management measures like banning shellfish harvest have been taken to prevent poisonings. Albeit necessary, these measures put many livelihoods at risk. For instance, the 1992 Pyrodinium red tide outbreak affected almost 40,000 fisherfolk, translating to an estimated economic loss of PhP 3.4 billion (2002 prices).4
HABs are a dire concern for many sectors of society — public health, economy, and livelihoods — and the development of early-warning tools can greatly improve our chances of mitigating them.
Clip: Dexter Dela Cruz for the Coastal Acidification Project 2
Training computers to forecast HABs
Methods to address HABs before or after they occur have been proposed and studied, but Dr. Yñiguez zeroes in on how to tell if a HAB is about to happen. From her first research assistantship under Dr. Rhodora Azanza to her work as a faculty member of UP MSI, Dr. Yñiguez has studied micro- and macroalgae, as well as their functions in marine ecosystems.
“For example, may potential na magkaroon ng fish kill. Then, within the next few days, the fish farmers can consider na, ‘Ah, okay, baka pwede na i-ahon ‘yung mga isda.’ Ma-prevent ‘yung likelihood na magkaroon ng fish kill because of the prediction, preventing losses. That’s early-warning mitigation.”
“It’s very interesting na, usually, diba hindi natin iniisip ang mga halaman. But then if they’re not there, we’re not here,” she says. “And everything else isn’t there either. I find [it] fascinating how they’re so important. Lalo na ‘yung mga phytoplankton and microalgae, ‘di naman pinapansin masyado.”
(“It’s very interesting that we usually don’t pay attention to plants. But, then, if they’re not there, we’re not here. And everything else isn’t there either. I find [it] fascinating how they’re so important. Especially the phytoplankton and microalgae, they’re not noticed much.”)
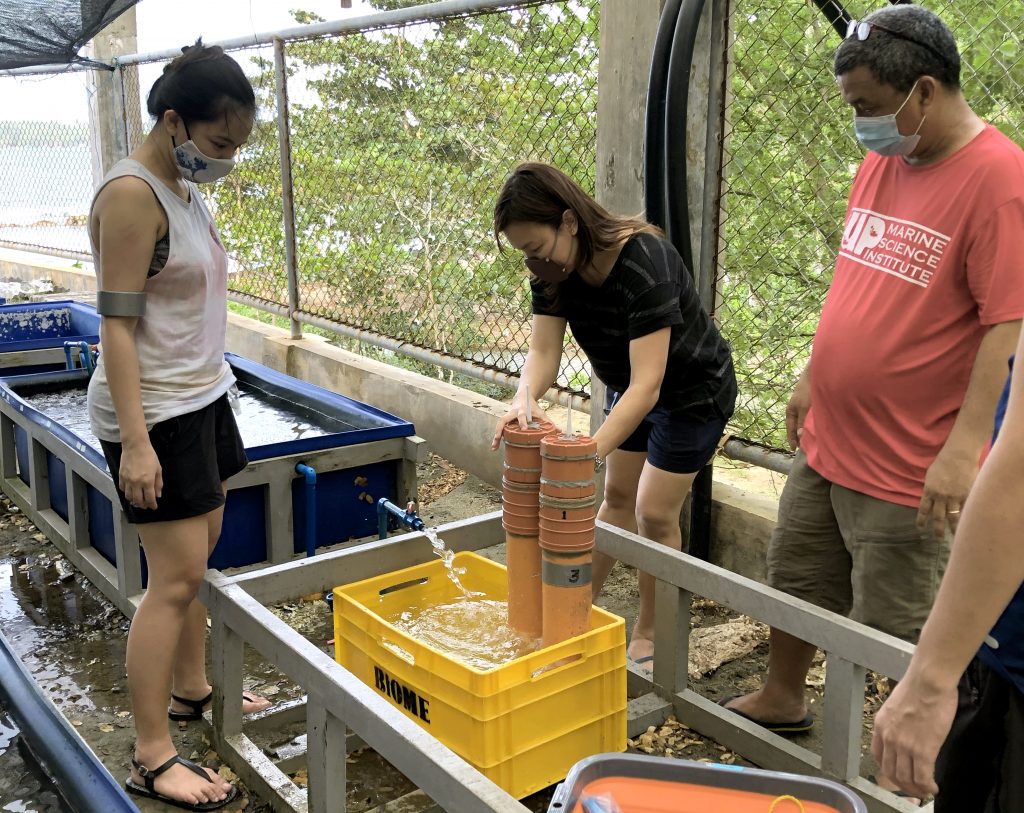
Photo: UP MSI BiOME lab
While algae are crucial to water-based ecosystems, too much of anything can be a bad thing. With her knowledge of algae and their behaviors, Dr. Yñiguez seeks to understand which environmental factors most likely have a relationship with the onset of HABs. Learning these factors helps her design algorithms to predict HABs, which should be set up in coastal or maritime locations ideally:
“For example, may potential na magkaroon ng fish kill. Then, within the next few days, the fish farmers can consider na, ‘Ah, okay, baka pwede na i-ahon ‘yung mga isda.’ Ma-prevent ‘yung likelihood na magkaroon ng fish kill because of the prediction, preventing losses. That’s early-warning mitigation.”
(“For example, a potential fish kill can happen. Then, within the next few days, the fish farmers can consider, ‘Ah, okay, maybe we can take out the fish.’ The likelihood of a fish kill can be prevented because of the prediction, preventing losses. That’s early-warning mitigation.”)
The random forest algorithm
The authors considered the physical (temperature and salinity) and chemical (dissolved oxygen and pH) properties of water, as well as its chlorophyll-a (Chl-a) levels. These factors were chosen based on the questions: are they relevant to HABs? Were the data obtained during a known HAB event? Can we acquire them in real-time?
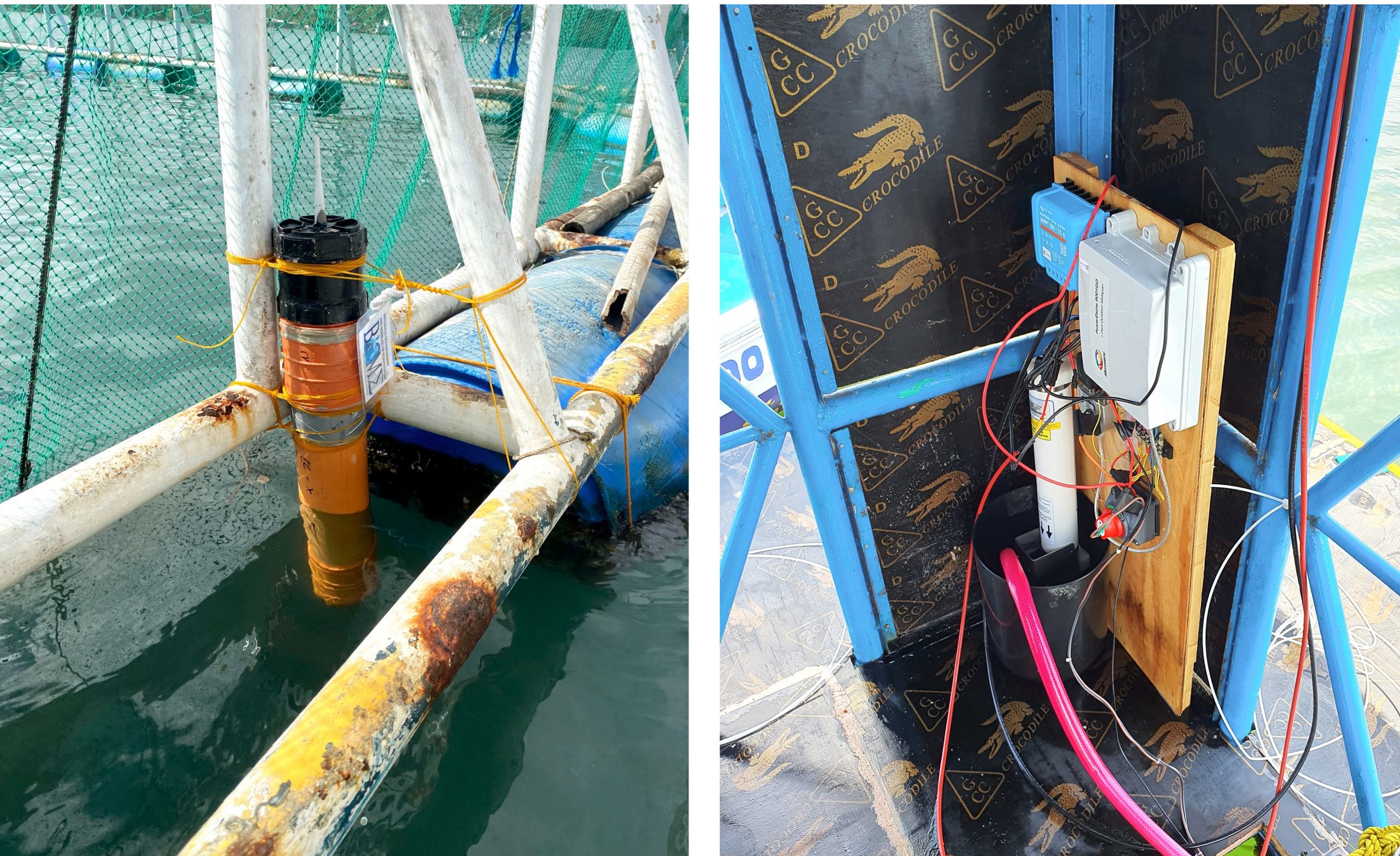
The data were cross-referenced with previous Bureau of Fisheries and Aquatic Resources (BFAR) reports, monitoring projects, and news articles to create a timeline of HAB events. Both fish kill events and toxic blooms were investigated.
We asked Dr. Yñiguez to let us in on how the algorithm works. “Whether you use Netflix, YouTube, or TikTok, you’re consuming specific content, tapos makikita mo may mga recommendations na. Bakit may recommendations na hawig sa mga nakita ko? Yung taste ko? Kung nanonood ka madalas ng BTS, dadami ‘yung associated videos with BTS or K-pop. That’s the concept of machine learning. You put in historical data tapos makikita [ng computer] anong pattern sa data na ‘yun. Based on that, ‘yung likelihood or types ng things that you watch na nakuha, magre-recommend na siya ng similar.
(“Whether you use Netflix, YouTube, or TikTok, you’re consuming specific content, then you notice the recommendations. Why are there recommendations based on what I see? On my taste? If you watch BTS often, the associated videos with BTS or K-pop will increase. That’s the concept of machine learning. You put in historical data then the [computer] sees the pattern in those data. Based on that, the likelihood or types of things that you watch, it will recommend something similar.)
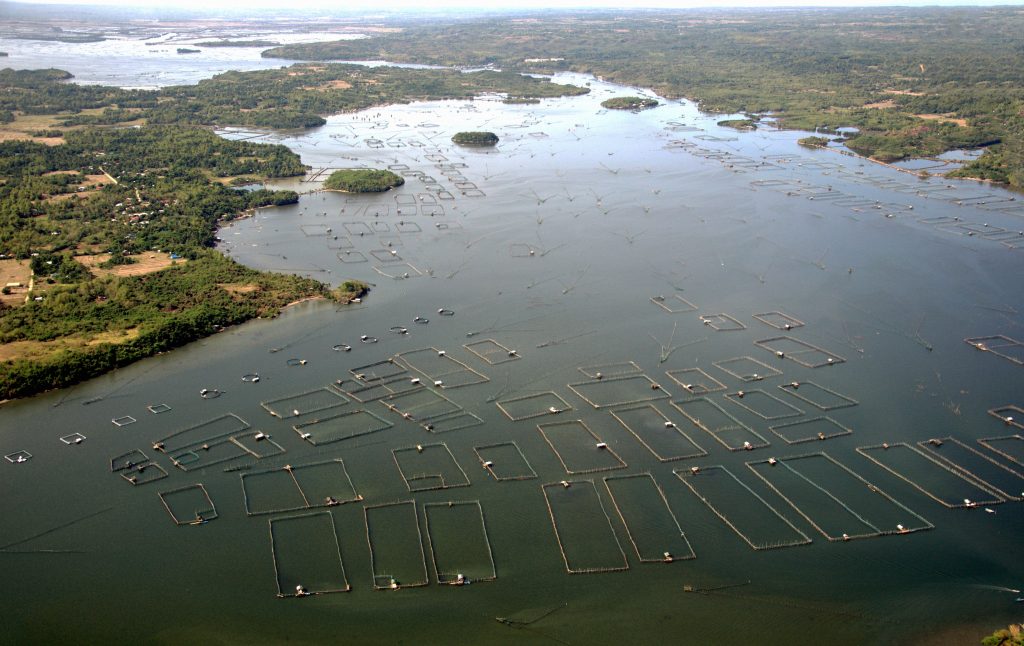
“Dito sa ginawa namin, ang historical data ay in the past several years na may HAB event sa Bolinao — may fish kill, may toxic bloom, may shellfish ban. Ano ‘yung mga environmental events associated doon sa mga ‘yun? The algorithm, then, gagawa ng mga trace of associated values and conditions at that time. Umuulit ‘yun nang umuulit na gumagawa siya ng trace until it approaches a threshold of accuracy para makuha niya nang tama ‘yung patterns. And then you have a separate data set na i-test natin kung napre-predict ba niya yung outcomes. Nagkaroon ba ng bloom o hindi based on an independent data set?”
(“In this work, the historical data include the past several years that there was a HAB event in Bolinao — a fish kill, toxic bloom, shellfish ban. What are the environmental events associated with those? The algorithm, then, produces traces of associated values and conditions at that time. The generation of traces repeats until it approaches a threshold of accuracy, so that it gets the patterns right. And then you have a separate data set to test if it can predict the outcomes. Did a bloom occur or not, based on an independent data set?”)
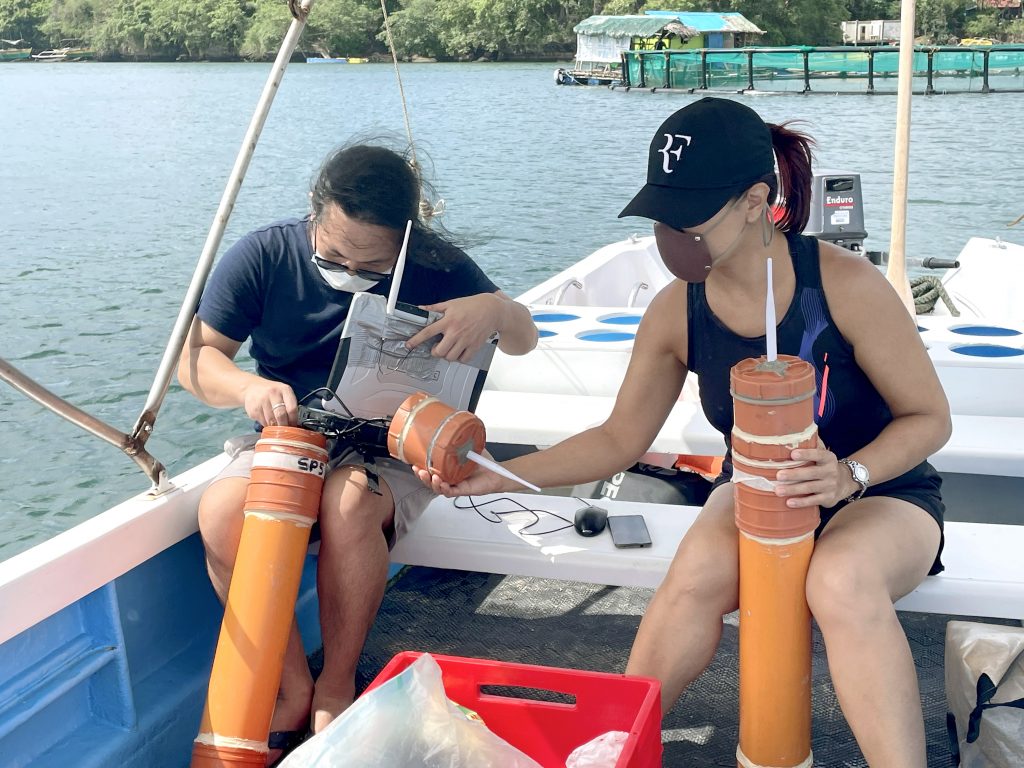
By continuously feeding data and validating the algorithm, a robust and dependable AI platform can be installed in regions that are vulnerable to HABs. The accuracy of the random forest algorithm was proven when it was able to predict an independent fish kill event on May 31, 2018.
Different factors or “predictive variables” worked for fish kills and toxic blooms, respectively. The algorithm predicts fish kills with 96.1% accuracy using dissolved oxygen (DO), temperature, and salinity. On the other hand, the forecast for toxic blooms is at 97.8% accuracy with DO, temperature, and Chl-a as key predictive variables. These are highly favorable results, but Dr. Yñiguez emphasizes that the model needs to be trained further using longer-term data.
Community-focused STEM advocacy
There are HAB hotspots all over the Philippines, so collaboration with different agencies is crucial to expediting the deployment of AI-based HABs forecast systems in communities. Currently, Dr. Yñiguez and her group are collaborating with BFAR, and they hope to work with other agencies like the Department of Environment and Natural Resources.
Apart from collaborating with agencies, involving fisherfolk and other members of the mariculture industry is equally important. If we build capacity within these communities, we can invite the primary stakeholders to participate directly in the monitoring and interpretation of data, giving them more autonomy when engaging in dialogues with their LGUs.
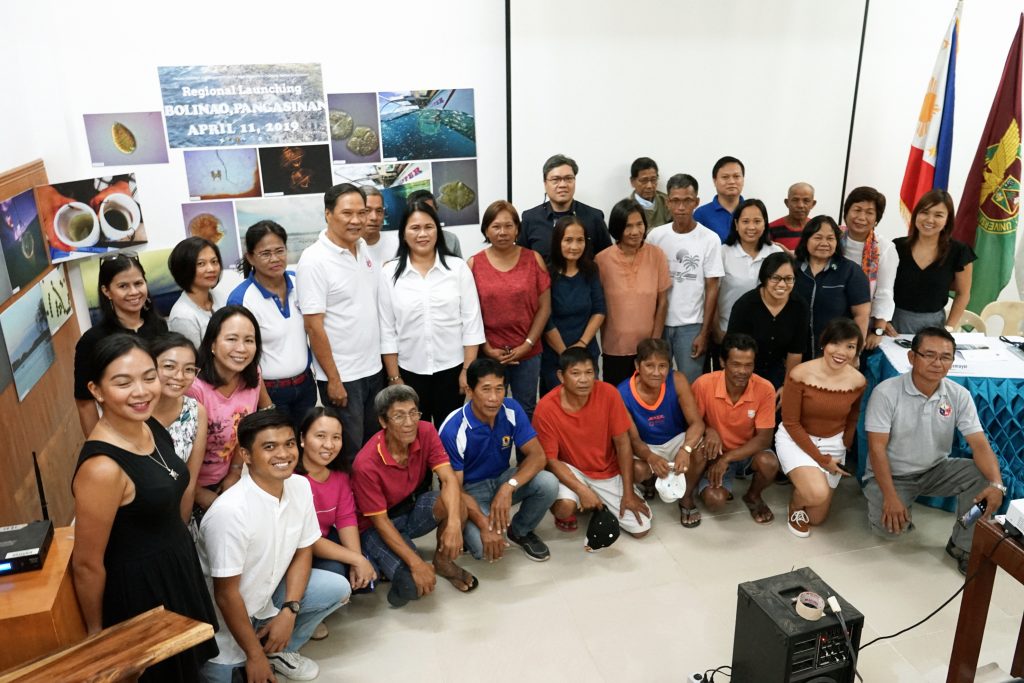
Dr. Yñiguez shares that her end goal with HABs research is to see real-time, early warning detection systems be implemented in the Philippines.
“Panaginip ko talaga ‘yung ma-push ang real-time automated detection. Dito kasi, [ang] sensors pa lang ay para sa physical [variables] pero I’d really like na kasama na talaga ‘yung images, like of the phytoplankton themselves in real-time, which is possible but super expensive. So we hope, sometime in the future, ay lower cost na siya.
(“My dream is for real-time automated detection to be pushed. Here, the sensors are only for physical [variables] but I’d really like to include images, like of the phytoplankton themselves in real-time, which is possible but super expensive. So we hope, sometime in the future, it’s going to come at a lower cost.)
“With that, we can have really great data and information and monitoring. Even if may COVID at ‘di ka makalabas, may data pa rin. Iyon ‘yung kulang dito sa Pilipinas, ‘yung long term na time series na information. How are you supposed to know ano ‘yung nangyayari sa site? Is it deteriorating, improving… How is climate change impacting it? If you don’t have that long term information, I’d really like [that] in different embayments in the Philippines, naka-set up ‘yung mga ganyang system at models that will make data and information just accessible to a lot of people, at magagamit siya for mariculture purposes or other fisheries na studies. It’s the future.”
(“With that, we can have really great data and information and monitoring. Even with COVID and restrictions on going out, there’s still data to acquire. That’s what the Philippines lacks, long term time series information. How are you supposed to know what’s happening to a site? Is it deteriorating, improving… How is climate change impacting it? If you don’t have that long term information, I’d really like [that] in different embayments in the Philippines, those systems and models are set up so that data and information will just be accessible to a lot of people, and these can be used for mariculture purposes or other fisheries research. It’s the future.”)
Involving fisherfolk and other members of the mariculture industry is equally important. If we build capacity within these communities, we can invite the primary stakeholders to participate directly in the monitoring and interpretation of data, giving them more autonomy when engaging in dialogues with their LGUs.
Curious about the real-time data and model forecasts that were developed in the article? Check out the HABHub site here!
Acknowledgments
The authors would like to acknowledge their collaborators in the development of the algorithms and applications: Dr. Giovanni Tapang and Alimuddin Arriesgado from the National Institute of Physics, UP Diliman; Dr. Cesar Villanoy, Dr. Charina Amedo-Repollo, Dr. Gil Jacinto, Dr. Ma. Lourdes San Diego-McGlone, Dr. Ma. Josefa Pante, Dr. Rhodora Azanza, Rodrigo Narod Eco, Rica Dungog, Paul Andrew Gravoso, Andalus Punongbayan, and Manuel Justin Custado from the UP MSI; and the Bureau of Fisheries and Aquatic Resources (BFAR) of Region 1. They would also like to thank the Department of Science and Technology Philippine Council for Agriculture, Aquatic and Natural Resources Research and Development (DOST-PCAARRD) for funding the project.
References:
1. Estudillo, R. A., & Gonzales, C. L. (1984). Red tides and paralytic shellfish poisoning in the Philippines. In Toxic Red Tides and Shellfish Toxicity in Southeast Asia: Proceedings of a Consultative Meeting held in Singapore 11-14 September 1984 (pp. 52-79). Marine Fisheries Research Department, Southeast Asian Fisheries Development Center.
2. Yñiguez, A. T., & Ottong, Z. J. (2019). Predicting fish kills and toxic blooms in an intensive mariculture site in the Philippines using a machine learning model. Science of The Total Environment, 136173. doi:10.1016/j.scitotenv.2019.136173
3. Hallegraeff, G. M., Anderson, D. M., Belin, C., Bottein, M. Y. D., Bresnan, E., Chinain, M., Enevoldsen, H., Iwataki, M., Karlson, B., McKenzie, C.H., Sunesen, I., Pitcher, G.C., Provoost, P., Richardson, A., Schweibold, L., Tester, P.A., Trainer, V.L., Yñiguez, A.T., & Zingone, A. (2021). Perceived global increase in algal blooms is attributable to intensified monitoring and emerging bloom impacts. Communications Earth & Environment, 2(1), 1-10.
4. Occurrence of Red Tide in Philippine Bay. Wepa-db.net. Retrieved 13 October 2021, from http://www.wepa-db.net/policies/measures/background/philippines/redtide.htm.
5. Basic Information on Red Tide and Harmful Algal Blooms (HABs). BFAR On-Line Information System. (2014). Retrieved 13 October 2021, from https://www.bfar.da.gov.ph/redtideinfo.jsp.
6. Causes and Ecosystem Impacts | Harmful Algal Blooms | CDC. CDC.gov. Retrieved 13 October 2021, from https://www.cdc.gov/habs/environment.html.
7. INQUIRER.net. (2021). Shellfish ban up in 18 areas. Retrieved 13 October 2021, from https://newsinfo.inquirer.net/1429921/shellfish-ban-up-in-18-areas.
8. https://newsinfo.inquirer.net/1450951/red-tide-warning-up-in-11-areas
9. Mhttps://www.pna.gov.ph/articles/1147231
10. https://www.philstar.com/nation/2021/06/10/2104484/algal-bloom-caused-greenish-water-taal-lake-denr-says
11. https://www.sciencedirect.com/science/article/abs/pii/S1568988320300561